Using natural language processing to improve everyday life
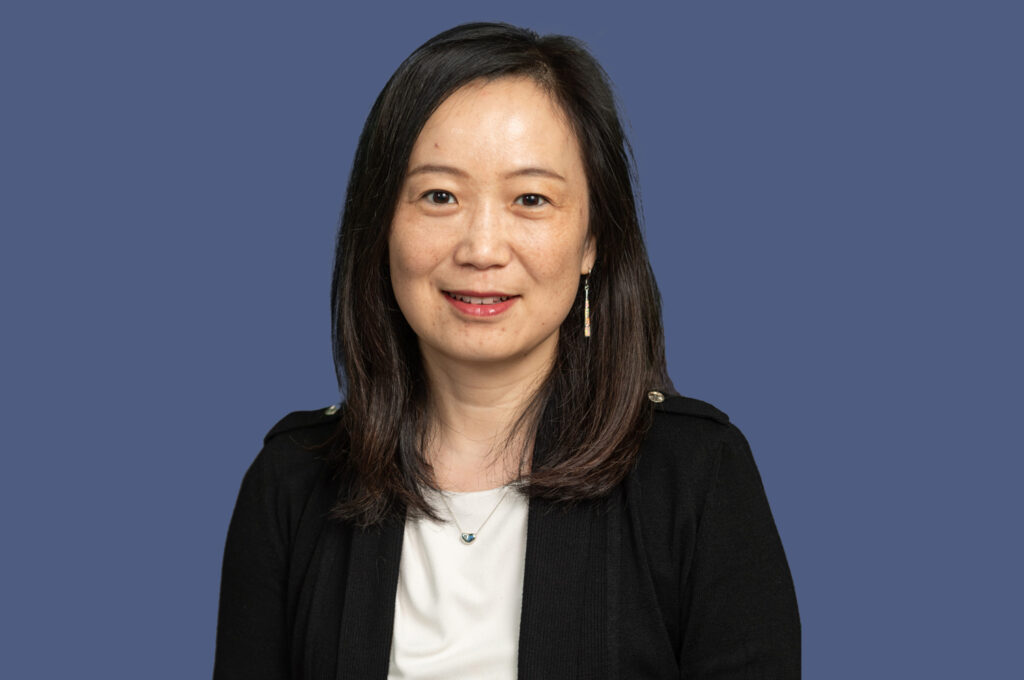
Joyce Y. Chai, professor of electrical engineering and computer science, College of Engineering, and associate Director of the Michigan Institute of Data Science and her colleagues have been seeking answers to complex questions using natural language processing and machine learning that may improve everyday life.
Some of the algorithms that they develop in their work are meant for tasks that machines may have little to no prior knowledge of. For example, to guide human users to gain a particular skill (e.g., building a special apparatus or even, “Tell me how to bake a cake”). A set of instructions based on the observation of what the user is doing, e.g., to correct mistakes or provide the next step, would be generated by Generative AI, or GenAI. The better the data and engineering behind the AI, the more useful the instructions will be.
“To enable machines to quickly learn and adapt to a new task, developers may give a few examples of recipe steps with both language instructions and video demonstrations. Machines can then (hopefully) guide users through the task by recognizing the right steps and generating relevant instructions using GenAI,” said Chai.
What are AI, machine learning, deep learning, and natural language processing?
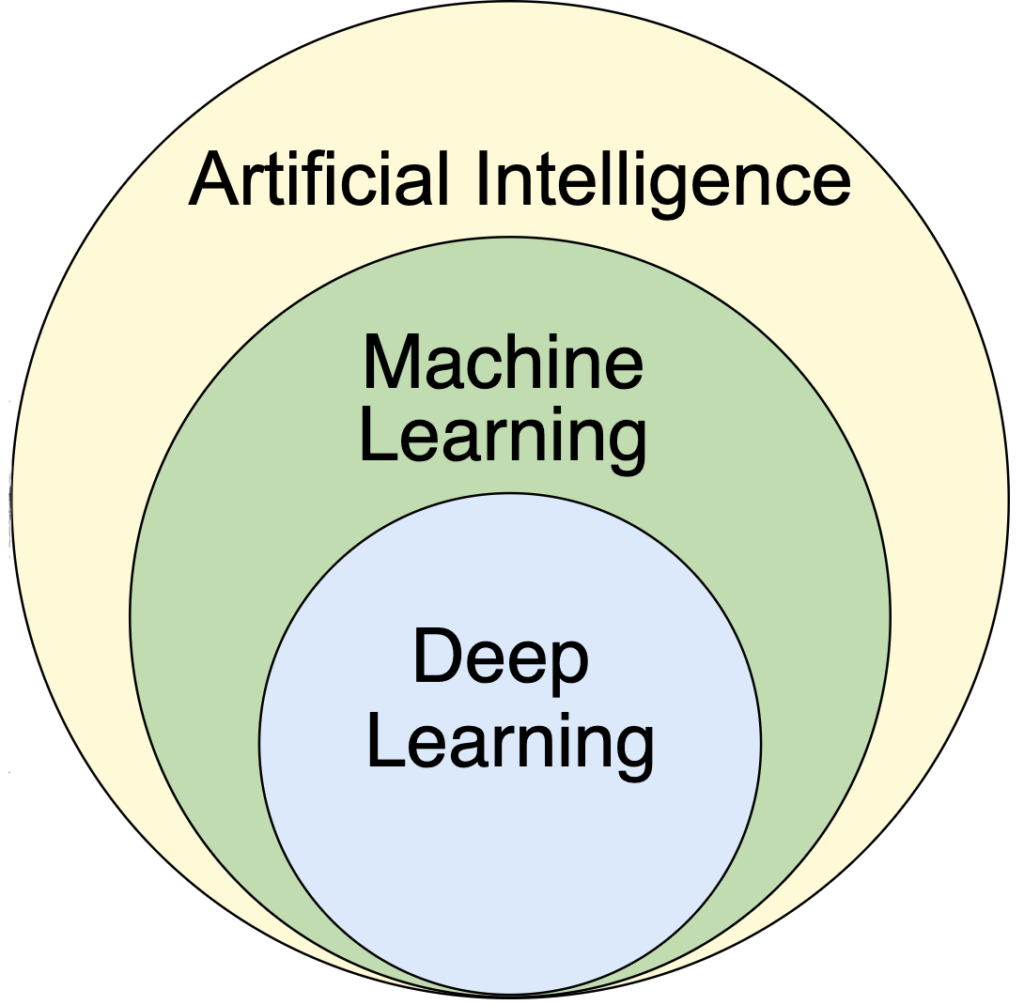
It might help to take a step back to understand AI, machine learning (ML), and deep learning at a high level. Both ML and deep learning are subsets of AI, as seen in the accompanying figure. Some natural language processing (NLP) tasks fall within the realm of deep learning. They all work together and build off of each other.
Artificial Intelligence, or AI, is a branch of computer science that attempts to simulate human intelligence with computers. It involves creating systems to perform tasks that usually need human intelligence, such as visual perception, speech recognition, decision-making, and translation between languages.
“NLP is a sub-area in AI, where DL/ML approaches are predominantly applied,” stated Chai.
Christopher Brown, a research data scientist, with ITS Advanced Research Computing (ARC) and a member of the ARC consulting team, explains that ML is a subfield of AI. Within ML, algorithms are used to generalize situations beyond those seen in training data and then complete tasks without further guidance from people. A good example is U-M GPT. The large language models (LLMs) accessible via U-M GPT are trained with millions of diverse examples. “The goal is to train the models to reliably predict, translate, or generate something.”
“Data is any information that can be formatted and fed into an algorithm that can be used for some task, including journal articles, chats, numbers, videos, audio, and texts,” said Brown, Algorithms can be trained to perform tasks using these real-world data.
Natural Language Processing is a branch of artificial intelligence that helps computers understand and generate human language in a way that is both meaningful and useful to humans. NLP teaches computers to understand languages and then respond so that humans can understand, and even accounting for when rich context language is used.
“NLP is highly interdisciplinary, and involves multiple fields, such as computer science, linguistics, philosophy, cognitive science, statistics, mathematics, etc.,” said Chai.
Examples of NLP are everywhere: when you ask Siri for directions, or when Google efficiently completes your half-typed query, or even when you get suggested replies in your email.
Ultimately NLP, along with AI, can be used to make interactions between humans and machines as natural and as easy as possible.
A lot of data is needed to train the models
Prof. Chai and her team use large language models, a lot of data, and computing resources. These models take longer to train and are harder to interpret. Brown says, “The state of the art, groundbreaking work tends to be in this area.”
Prof. Chai uses deep learning algorithms that make predictions about what the next part of the task or conversation is. “For example, they use deep learning and the transformer architecture to enable embodied agents to learn how new words are connected to the physical environment, to follow human language instructions, and to collaborate with humans to come up with a shared plan,” Brown explains.
The technology that supports this work
To accomplish her work, Prof. Chai uses the Great Lakes High-Performance Computing Cluster and Turbo Research Storage, both of which are managed by U-M’s Advanced Research Computing Group (ARC) in Information and Technology Services. She has 16 GPUs on Great Lakes at the ready, with the option to use more at any given time.
A GPU, or Graphics Processing Unit, is a piece of computer equipment that is good at displaying pictures, animations, and videos on your screen. The GPU is especially adept at quickly creating and manipulating images. Traditionally, GPUs were used for video games and professional design software where detailed graphics were necessary. But more recently, researchers including Prof. Chai discovered that GPUs are also good at handling many simple tasks at the same time. This includes tasks like scientific simulations and AI training where a lot of calculations need to be done in parallel (which is perfect for training large language models).
“GPUs are popular for deep learning, and we will continue to get more and better GPUs in the future. There is a demand, and we will continue supporting this technology so that deep learning can continue to grow,” said Brock Palen, ITS Advanced Research Computing director.
Chai and her team also leveraged 29 terabytes of the Turbo Research Storage service at ARC. NLP benefits from the high-capacity, reliable, secure, and fast storage solution. Turbo enables investigators across the university to store and access data needed for their research via Great Lakes.
Great Lakes HPC in the classroom
ARC offers classroom use of high-performance computing cluster resources on the Great Lakes High-Performance Computing Cluster.
Prof. Chai regularly leverages this resource. “Over 300 students have benefited from this experience. We have homework that requires the use of the Great Lakes, e.g., having students learn how to conduct experiments in a managed job-scheduling system like SLURM. This will benefit them in the future if they engage in any compute-intensive R&D (research and development).
“For my NLP class, I request Great Lakes access for my students so they have the ability to develop some meaningful final projects. We also use the Great Lakes HPC resources to study the reproducibility for NLP beginners,” said Chai. A gallery is available for many of the student projects.
The UMRCP defrays costs
The U-M Research Computing Package is a set of cost-sharing allocations offered by ITS ARC, and they are available upon request. Other units offer additional cost-sharing to researchers. Chai said, “We typically use the nodes owned by my group for research projects that require intensive, large-scale GPU model training. We use the UMRCP for less intensive tasks, thereby extending the budgetary impact of the allocations.”